AI & ML Latest Trends
Artificial intelligence has become a motorist of technological progress in our increasingly digital data-driven world. This is justified by the fact that AI is expected to see an annual growth rate of 37.3% from 2023 to 2030.
Technological inventions and the latest innovation in artificial intelligence are leading to significant changes in the nature of work for nearly a quarter of all workers in developed countries. That means millions of people must acclimatize to the new demands and chops in time to keep their jobs and inflows, or the application of additional AI technologies will simply replace them.
Deploying the right AI technologies in your organization can help you automate routine tasks, gain insights through data analytics, and engage better with customers. Given the broad spectrum of AI’s applications in organizations, it’s no surprise that AI Specialist is the top-rated job on the LinkedIn Emerging Jobs Report in 2020. With an annual growth rate of 74% for this position, every industry is clamoring for AI talent to first devise a strategic plan for AI applications.
$15.7 Trillion Potential contribution to the global economy by 2030 from AI SOURCE: PWC | $93.5 Billion Value of the global AI market in 2021, projected to grow at a CAGR of 38.1% through 2030 SOURCE: GRAND VIEW RESEARCH |
Increased Focus on AI Governance
As the AI powered technologies becomes more pervasive and powerful, there is an increased need for AI governance, which is the set of policies, principles, and practices that aim to ensure the ethical, safe, and responsible use of AI. It mainly revolves around two aspects:
New AI Regulations and Data Quality: As AI applications involve the collection, processing, and sharing of large amounts of data, there is a need for new regulations and standards to ensure the quality, accuracy, and privacy of data, as well as the compliance and accountability of AI systems and actors. For example, the European Union has proposed the Artificial Intelligence Act, which is a legal framework that sets out the rules and requirements for the development and deployment of AI systems in the EU.
Balancing between Automated and Human Labor: As AI automates various tasks and processes, there is a need to balance between the benefits and risks of automation. While automation enhances efficiency, productivity, and quality, it also poses challenges such as worker displacement, reduced human agency, and the potential for social and economic inequalities. Navigating this balance is essential for the evolving landscape of human resources in an AI-driven era.
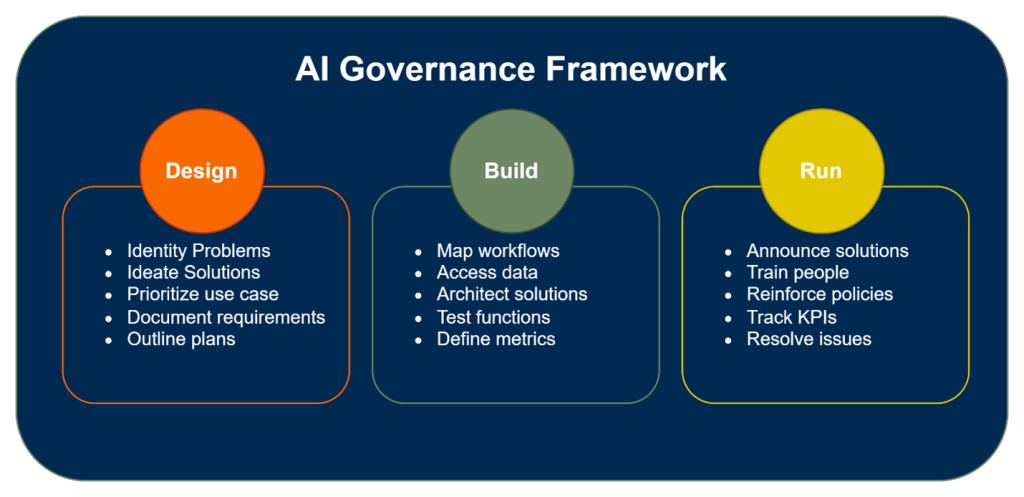
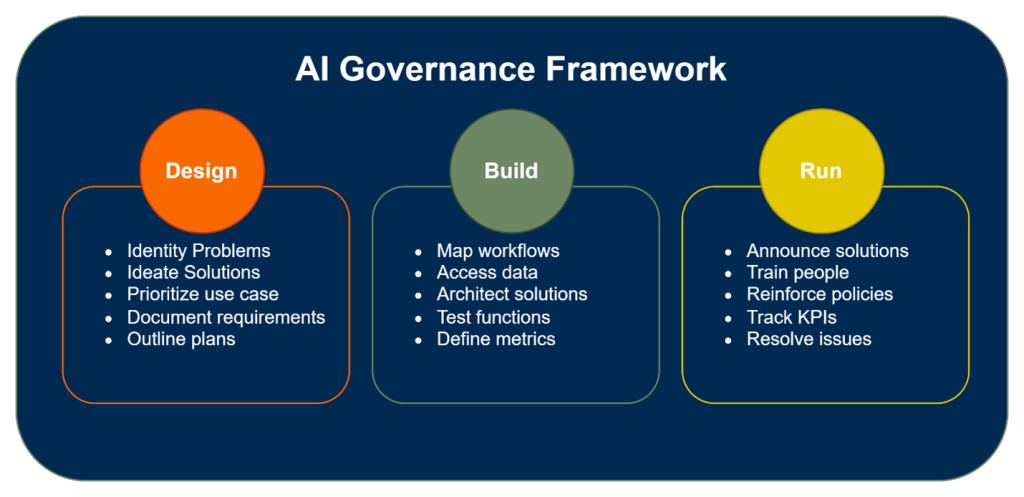
Ethical and Explainable AI
The topics of ethics and explainability in AI and ML are in the spotlight with more and more organizations considering the incorporation of ethical considerations and transparency mechanisms into artificial intelligence systems. This involves addressing biases in algorithms, preventing discrimination, and considering the social impact of AI applications.
AI Explainability refers to the ability of an AI system to provide understandable and transparent explanations for its decisions and actions. This is crucial in scenarios where AI systems impact human lives, such as healthcare, finance, and criminal justice. Transparent AI systems not only build trust, but also enable users to comprehend and contest decisions made by the AI.
While OpenAI’s ChatGPT, Google’s Bard and other similar AI tools have brought AI to the public, organizations have been using AI and Machine Learning (AI/ML) for several decades. Recent AI technologies based on Large Language Models will further boost the use of Generative AI in newer areas to increase productivity across various functional areas. Generative AI is also likely to be integrated with other technologies like Business Intelligence (BI), Robotic Process Automation (RPA) and functional areas like HR, Marketing, Sales and Legal.
However, until now, organizations have been developing and deploying AI/ML models under the radar without any oversight from regulators or the public in general. With AI now in the glaring spotlight, all these models will come under scrutiny from public, consumer groups and state/local/federal regulators.
Setup an AI/ML Governance which can work hand in hand with existing Data Governance or Business Intelligence Competency Center (BICC)organization if already in place. This does not have to be a new entity or bureaucracy. Charters of existing governance structures could be amended to include AI/ML Governance.
Identify Data Sets Used – For each model, it is critical to understand the data sets used to train the models. At the very minimum organizations will need to document any public data or third-party data with a clear trail of ownership rights to make sure there are no legal issues with regard to copyright laws.
Explainability – Organizations will need to ensure that the AI/ML models that will be used for customer experience, communication or interaction through chatbots or otherwise do not have any bias that violate any local, state, federal or international laws.
Label Content – It will be obligatory to label content created by AI/ML models especially when it is used a part of interaction through Chatbots.
Audit Framework – The AI/ML models should be regularly audited to ensure that they continue to comply with regulations as they evolve over time.
Reinforcement Learning for Optimizing Decision-Making
In machine learning, three paradigms exist: supervised learning, unsupervised learning, and reinforcement learning. Reinforcement learning involves the ML system learning through direct experiences with its environment, where the environment utilizes a reward/punishment system to assign value to the observations made by the ML system.
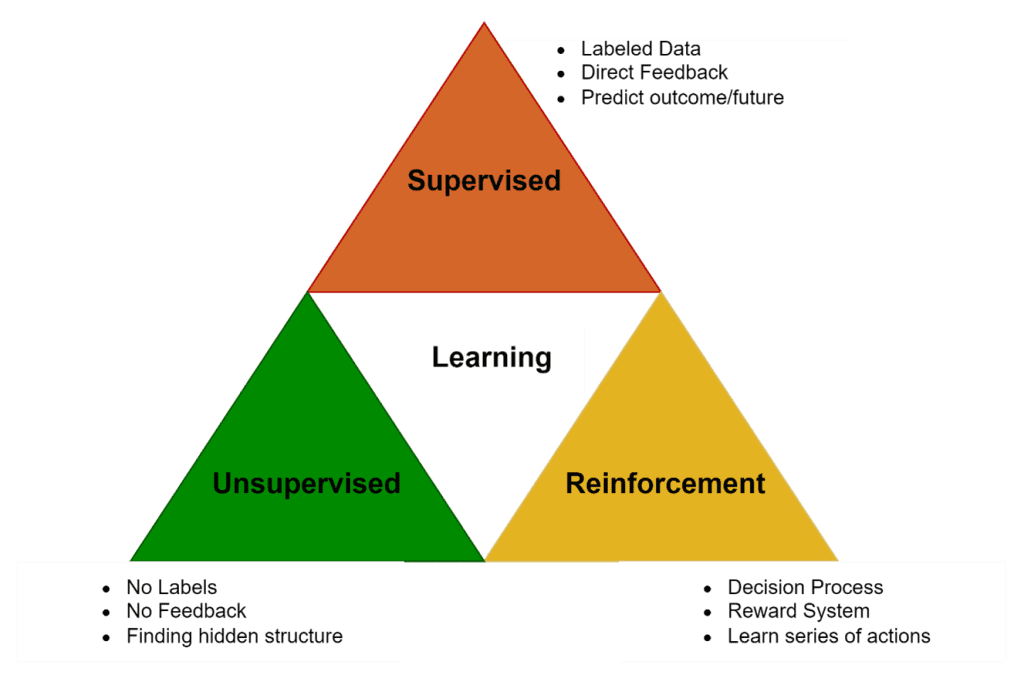
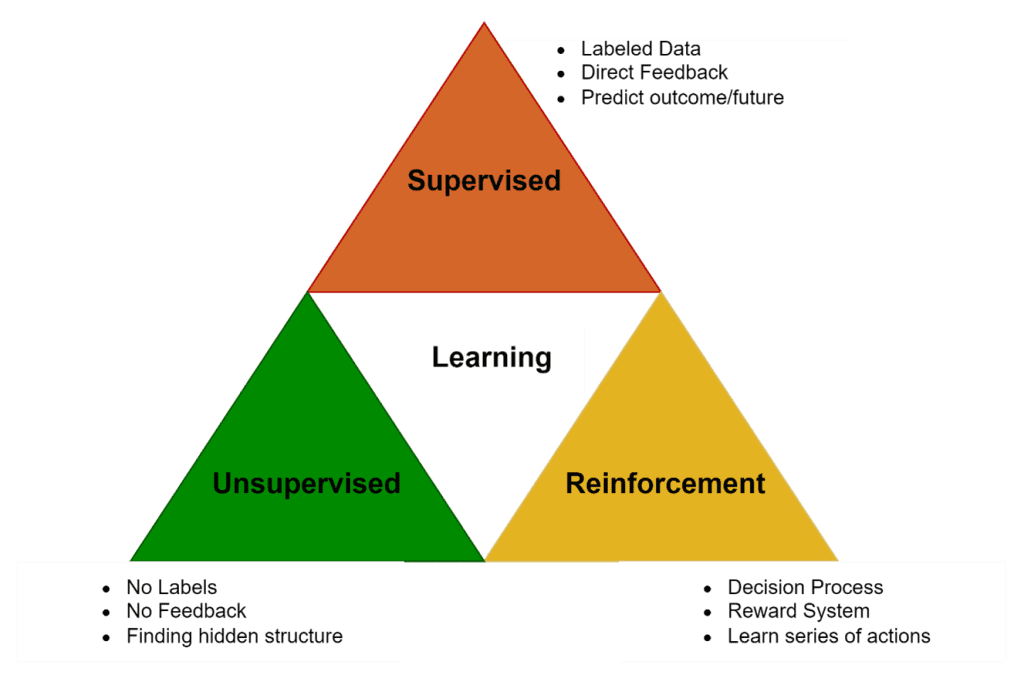
Low-Code/No-Code Machine Learning for Cost Cutting
Low-code and no-code ML platforms are useful for simple ML projects like retail profits, dynamic pricing, and employee retention rates. However, the development of advanced and multifunctional projects with significant business value usually is impossible without the ML engineers’ involvement. It’s obvious that developing advanced ML projects requires a human touch to perform data analysis correctly and efficiently.
AI-based Cybersecurity Improvements
AI is also playing a vital role in enhancing the cybersecurity of various systems and networks, by enabling new ways of detecting, preventing, and responding to cyberattacks. Some of the applications of AI in cybersecurity are:
AI-based Solution Development: This involves using AI techniques, such as machine learning, deep learning, or reinforcement learning, to develop and deploy new and effective cybersecurity solutions, like antivirus, firewall, or encryption software, that can learn from data, adapt to changes, and improve over time.
AI-driven Experimentation: This involves using AI techniques, such as genetic algorithms, swarm intelligence, or adversarial learning, to create and test new and innovative cybersecurity scenarios, such as attack vectors, defense strategies, or counter measures, that can simulate real-world situations, evaluate the performance, and optimize the outcome.
Journey towards Full-fledged AGI – Artificial General Intelligence
AGI refers to machines that can perform any intellectual task that humans can, or even surpass them. While we have made remarkable progress in narrow AI, that is specialized for specific domains, such as image recognition, natural language processing, or chess playing, we are still far from achieving the generality and versatility of human intelligence.
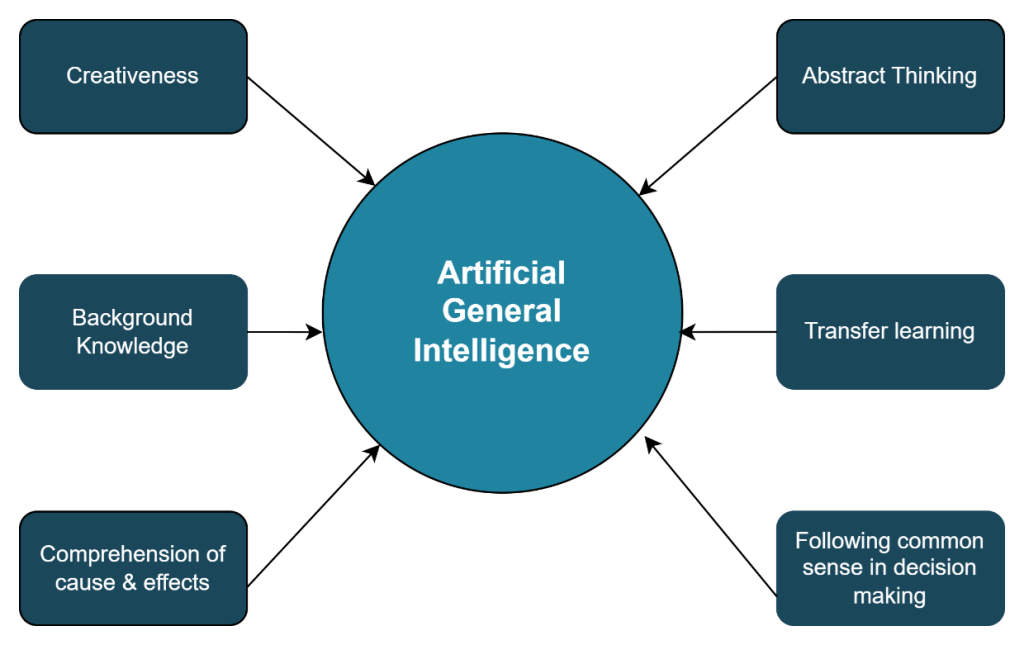
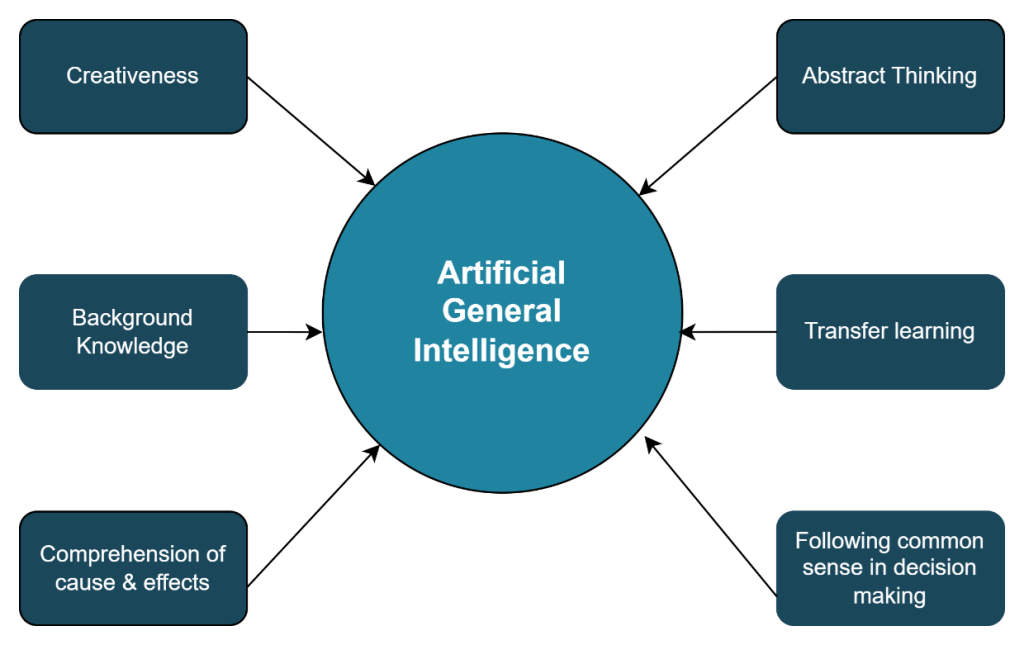
One of the main challenges in developing AGI is the availability and quality of data. Moreover, these systems tend to learn superficial patterns and correlations, rather than deep causal and conceptual understanding. To overcome these limitations, some researchers have proposed two promising directions:
Democratization for Cloud GPUs: One of the key drivers of AI innovation is the availability and accessibility of computing resources, like graphics processing units (GPUs), which enable fast and efficient processing of large amounts of data. Cloud GPUs are a service that allows users to rent GPUs from cloud providers, such as Amazon Web Services, Google Cloud Platform, or Microsoft Azure, and use them for various AI applications, such as training deep neural networks, running simulations, or performing data analysis. Cloud GPUs offer several benefits, like scalability, flexibility, cost-effectiveness, and ease of use. They also enable the democratization of AI by lowering the barriers to entry and allowing more people and organizations to access and leverage AI technologies.
Self-teaching Models to Create Human-Like Intelligent Results: Another trend in AI is the development of self-teaching models, which are capable of learning from their own experiences, without requiring explicit supervision or guidance from humans. Self-teaching models can achieve human-like or even superhuman performance on various tasks, such as playing games, generating natural language, or recognizing images. Some examples of self-teaching models are AlphaGo, which learned to play the game of Go by playing against itself, Chat GPT based on GPT-3 which learned to generate natural language by analyzing a large corpus of text, and DALL-E, which learned to generate images from text descriptions by using a multimodal dataset. Self-teaching models can create novel and intelligent results, but they also pose challenges, such as ensuring their reliability, explainability, and alignment with human values.
Conclusion
These trends show the importance and impact of AI and ML for the future of technology, society, and humanity. However, the integration of AI-powered technologies is not without its set of challenges and risks. Therefore, it is imperative to approach their use with awareness, responsibility, and ethical considerations. Moreover, preparing for an AI-powered future demands adaptability and resilience, as it is set to revolutionize the way we work, live, and interact. Balancing the potential benefits with ethical considerations is crucial in navigating the evolving landscape of AI-powered technologies.